Artificial Intelligence (AI) is transforming the world at an unprecedented pace, offering significant benefits, and creating new opportunities across various sectors. However, the rapid adoption of AI technologies also presents a myriad of ethical and legal challenges, particularly in the realm of data protection and privacy. Therefore, the General Data Protection Regulation (GDPR), a regulation directly effective in each EU member state, has become a critical consideration for organizations leveraging AI technologies. This article explores the intersection of GDPR compliance and the usage of AI, highlighting the ethical and legal challenges and providing insights on how to navigate this complex landscape.
Understanding the Basic Principles of AI and GDPR and Their Mutual Intersection
AI refers to systems that learn from data, identify patterns, and make decisions tending to be without, or with minimal, human intervention. These systems can range from simple rule-based systems to complex machine learning and deep learning models.
Regardless of whether we are discussing AI in the form of neural networks, machine learning, optimization, genetic algorithms, or any other type, they all share a common characteristic – they process data. AI systems often rely on large volumes of data, including personal data, to train and improve their models.
Although various types of data might be subject to different legal frameworks aiming at their protection and safety, the GDPR lays down rules relating to the protection of natural persons with regard to the processing of personal data and rules relating to the free movement of personal data (Article 1 of the GDPR). Hence, it can be also concluded that the GDPR represents a comprehensive data protection law affecting organizations that process the personal data of EU citizens on a worldwide scale.
Speaking about the point of concurrence, for both AI and GDPR, the light must be focused on what kind of data are being processed or used by AI and how this procession is made when it comes to personal data falling within the scope given by the GDPR. The data-driven nature of AI brings it squarely within the purview of the GDPR. However, the complexity and opacity (the limited ability of the human mind to understand how certain AI systems operate) of some AI systems, particularly those based on machine learning, can make it challenging to ensure and demonstrate compliance of such systems with GDPR.
Above all, the GDPR stipulates principles on data processing, namely: lawfulness, fairness, transparency, data minimization, accuracy, purpose and storage limitation, integrity, confidentiality, and accountability. Nonetheless, it also provides individuals with rights, such as the right to access and information, rectify, erase, restrict processing, data portability, object, and rights related to automated decision-making, including profiling. These standards and conditions are challenged by AI processing.
All principles given by the GDPR are bound together and are interrelated when it comes to processing data and obeying the law to provide the data subject with an adequate full range of legally granted rights.
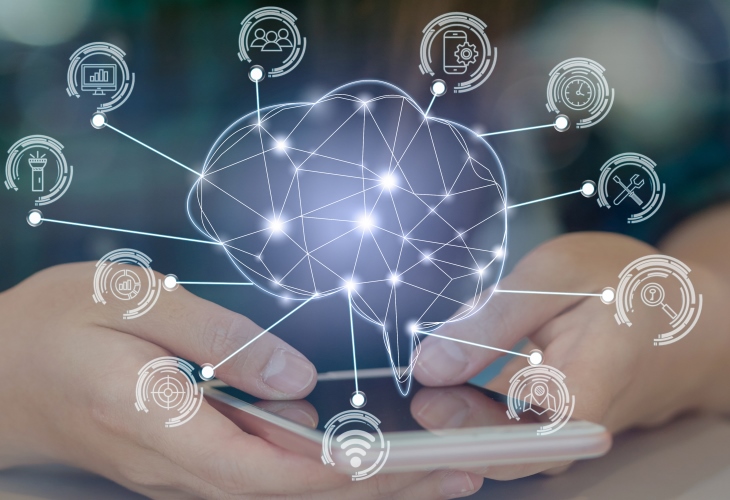
Transparency and Automated Individual Decision- Making, Including Profiling
One of the key challenges is the ‘black box’ nature of the majority of the AI systems, which makes it difficult to understand not only how the decision they made is adopted, but also their complexity, autonomy, and lack of transparency which makes it extremely difficult to assort the AI within most of the common legal institutes (following not only data procession).
The conflict arising from the lack of transparency in AI systems necessitates further action to align with the GDPR’s principle of transparency. But is it possible to bring reasonably clear and understandable information about processing personal data when it comes to AI systems?
That is the question that needs to be answered. Looking at it through some general software I would say rather impossible than even close to yes. Even the data controller does not know how to answer the question of transparency as it is based on machine learning – when it goes to learn and how it works the algorithm, when it learns to be, and to what percentile correct. What we have to look into much deeper is not only how the AI system is working itself but also the character of the data it is processing and the result of the processing system itself and how that might or not affect the data subject.
The GDPR provides individuals with a ‘right of not being subject to a decision based solely on automated processing, including profiling, which produces legal effects concerning, or similarly significantly affects him or her’ being even more strict when it comes to specific categories of personal data. This would be crucial to any automated decision-making which represents the possibility of making decisions purely by technological means based on any type of data.
And it is optional whether the automated decision-making is the result of an assessment of data provided by the individuals themselves or of observed, derived, or inferred data. The significance is only that it is a decision without human intervention and might have a defined effect on someone who would object to the result.
The generated information about certain characteristics or predispositions can influence, for example, an employer who decides to use such results to reject an applicant’s application for employment. Although the entered input parameters appear neutral on the outside, it cannot be ruled out with certainty that in mutual combinations they are essentially protected characteristics that should not be considered at all due to respect for the principle of equal treatment.
The Regulation does not a priori prohibit decision-making based on automated processing but grants the data subject the right not to be subject to a decision based solely on such processing, which has legal effects that concern them or similarly significantly affect them (Article 22(1) of the GDPR). However, it is important that such a decision is predictable and that it is carried out based on legally compliant rules. This brings us back to the challenges addressed by the AI systems.
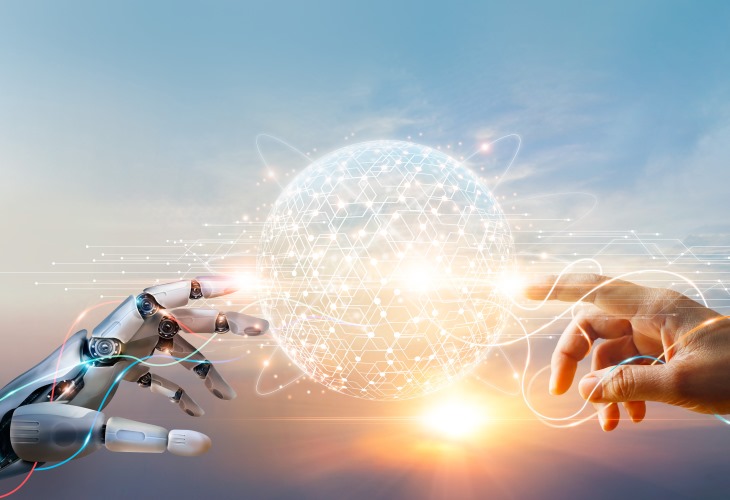
The AI Act
The proposed wording of an EU regulatory framework on artificial intelligence could provide some guidance to help us navigate in the right direction. The draft AI Act is the first comprehensive EU legislation to regulate AI and address its potential harms aiming to promote the uptake of AI and encounter the risks associated with the technology. The AI Act follows a risk-based approach and lays down obligations for providers and those deploying AI systems, depending on the level of risk that such a system may pose.
The given framework distinguishes between i) an unacceptable risk; ii) a high risk, and iii) a low or minimal risk AI system. AI systems with an unacceptable level of risk to human safety should be banned, according to the proposal. The prohibitions apply to practices that have a significant potential to manipulate persons through subliminal techniques outside of their awareness or to exploit the vulnerabilities of certain vulnerable groups, such as children to significantly disrupt their behavior in a way that can cause them psychological or physical harm (these include, for example, systems used to evaluate the so-called social scoring, i.e. classifying people based on their social behavior or personal characteristics).
The designation as high-risk in the sense of the drafted regulation does not depend only on the function performed by the artificial intelligence system, but also on the specific purpose and ways in which this system is to be used.
In addition to this, generative AI systems based on models such as ChatGPT (interactions or content creation) by design may, under certain circumstances, pose a particular fraud risk associated with impersonation or outright fraud, regardless of whether they are classified as high-risk or not. This is precisely why transparency requirements (disclosing that the content was generated by AI and similarly for so-called “deep fakes”) and ensuring guarantees against the generation of illegal content are introduced for them.
All these principles incorporated within the AI Act tend to the same purpose. Organizations willing to benefit given by AI advantages must be able to explain how their AI systems make decisions to be capable of granting certain levels of protection requested by law when it comes to certain risk levels of AI systems. There is no doubt this can be challenging for complex machine-learning models. Further to the obligation given by the AI Act, organizations can adopt explainable AI techniques to make their AI systems more transparent and understandable.
They can also provide clear and accessible information about their use of AI and its impact on individuals.
Fairness and Non-Discrimination
AI systems can inadvertently lead to unfair or discriminatory outcomes if they are trained on biased data or if their learning algorithms are not properly designed or controlled. Decisions made by computers after a machine learning process may be considered unfair if they were based on variables considered sensitive, such as religion, gender, sexual orientation, race, disability, or any kind of information that their subject protects to come to the public (financial status, etc.).
A good example might be the procession of data on employees’ spouses by the employer. Even though sometimes such information to be processed is inevitable (for instance to apply for certain tax or social deductions or further related bonuses) it shall not be processed in a way that the employer might take discriminatory measures against the employee based on their sexual orientation.
Furthermore, we can hear everywhere that the data subject has to have the right to object to biased decision-making when it comes to processing their financial data. Such outcomes can violate the GDPR’s principle of fairness and its provisions on automated decision-making, including profiling.
To avoid such violations organizations can use fairnessaware machine learning techniques to prevent unfair or discriminatory outcomes. Nevertheless, in recent years, tech companies have developed tools and manuals for detecting and reducing bias in machine learning, it might be the Gordian knot. Hence, our recommendation (based on GDPR best practices) for organizations would be to include human factors and conduct regular audits and impact assessments which might represent big help when it comes to identifying and mitigating potential biases or unfairness in their AI systems.
Data Accuracy, Minimization, and Purpose and Storage Limitation
As it was said already AI systems often benefit from huge data resources. The more data the AI system has to be fed with more complex results it can provide. Hence it is undoubtedly correct that a range of data can improve their performance and accuracy. However, this can conflict with the GDPR’s principles of data minimization and purpose limitation, which require organizations to collect only the data necessary for a specific given purpose (at the time of defining the purpose of processing and at the time of the processing itself) not speaking about the limitation on time of the processing – storage limitation. These limits AI systems to strictly following legally given periods for any processing or using data or following the exception given by the law.
Following what was said already, AI systems may often use provided data to infer or even create new content or even further data to process. There have been multiple cases worldwide when the AI system had been hallucinating, i.e. generated output or perceived patterns that did not correspond to reality, or made logical sense in a given context. Such processing would be considered (apart from archiving, statistics, or some research purposes) as valueless and represent a high risk of affecting individuals. Other obligations of the controller, such as the right to rectify, are also bound by this principle.
The organization processing data must consider risks of varying probability and severity for the rights and freedoms of data subjects that arise when processing personal data. When considering the level of risk, it is advisable to follow proven methods that serve to manage risks, identify them, determine the level of risk, and define the potential threat and vulnerability of the system. Such a risk management system has to be understood as a continuous iterative process throughout the life cycle, especially in the case of a high-risk AI system, which requires regular systematic updating.
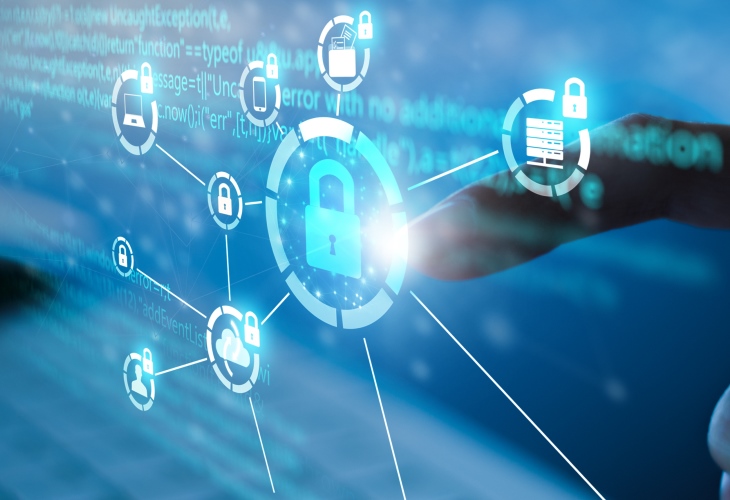
Integrity, Confidentiality, and Accountability
The GDPR states that the controller is obliged to secure the data against any lawful access or data loss. Organizations can incorporate data protection considerations into the design (e.g. data protection by design measures) and operation of their AI systems. This can involve techniques such as differential privacy (learn general trends, not individual’s private info), which adds noise to data to prevent the identification of individuals, and federated learning, which trains AI models on decentralized data (train the algorithm via multiple independent sessions, each using its dataset).
Further protection measures of data by default, by the provision of user service settings that would be data protection-friendly, may represent a good start for GDPR compliance – only the necessary data would be gathered for each specific purpose of the processing. Equally significant is the obligation given by GDPR for data accountability which means that any organization processing personal data is obliged not only to implement appropriate and effective measures but simultaneously be able to demonstrate that processing activities they run are compliant with the regulation.
The GDPR introduces a framework, according to which adherence to, for instance, specific Codes of conduct should be considered as compliance with the regulation (besides, any such statement of compliance would not exempt anyone from the jurisdiction control authorities it can just serve as guidelines).
But having in mind all of what was stated herein, emphasizing the black box principle of AI stands above the edge of any compliance tick box that has been given yet.
Navigating the Challenges: Conclusion
Despite the challenges at the intersection of AI and GDPR, there are strategies and best practices that organizations can employ to navigate this complex landscape. The friction points between AI and GDPR present not only challenges but also opportunities. It is essential that these two elements work in synergy for the collective benefit.
In the fast-paced realm of AI, regulatory structures are essential in setting guidelines and mitigating possible risks. It is only through adaptable regulations, which can keep pace with the constant evolution of AI and are supported by adequate enforcement capabilities, that we can safeguard associated rights. To be ready for such challenges, organizations (not only those who fell within legal obligation) should consider having a Data Protection Officer (DPO) with expert proficiency and a management team that is mindful of cybersecurity. These two areas are crucial for maintaining compliance in this dynamic environment.
Only by gaining a deep understanding of these issues and implementing appropriate strategies and best practices, such as ISO 42001, organizations can harness the power of AI while maintaining GDPR compliance and respecting individuals’ rights and freedoms. As AI continues to evolve rapidly, it becomes increasingly important for every single organization to stay informed and proactive in addressing emerging ethical and legal challenges throughout its lifecycle.