In this piece, we will explore how AI has revolutionized cybersecurity risk management. We will look at how AI is applied in a range of cybersecurity tasks and processes, all in order to create advanced and efficient response mechanisms to deal with the latest threats.
Likewise, we will highlight how AI has helped improve traditional cybersecurity methods, while also considering the integration challenges in terms of existing risk management frameworks. From ethical considerations to potential AI bias, we will provide a balanced overview of how AI is helping to shape the future of cybersecurity.
What Are the Current Limitations of Threat Detection?
Threat detection, both in terms of malware and existing network security analysis techniques leaves organizations and their data vulnerable to a range of cyber-attacks.
Many systems still rely on signature-based techniques, detecting malware based on the individual properties of the software.
However, malware is becoming increasingly more sophisticated, and this traditional method is considered no longer fit for purpose. For example, this method is not effective at detecting unknown malware, polymorphic malware, metamorphic malware, malware packing techniques, and code obfuscation.
These limitations also extend to wireless networks as they are particularly vulnerable to encryption attacks and eavesdropping techniques. Many existing threat detection models have low accuracy. This is because a truly effective system requires significant computational resources to scan the huge amount of data needed to identify new and existing threats.
Although deep learning-based classifiers improve on signature-based techniques, they can still be flawed.
The fact that modern malware can easily spread to mobile and IoT devices, can make it very difficult to detect. This is why it should be combined with advanced artificial (AI) and machine learning (ML) algorithms to provide a comprehensive detection strategy. However, for many organizations, integrating technology such as artificial neural networks, big data analysis, and complex machine learning algorithms is a significant challenge.
As a result of this investment, the cybersecurity market is projected to grow by 10.48% from 2015 to $10.5 trillion annually by 2025.
Integrating AI-Powered Cybersecurity: The Challenges
There are nine key challenges when integrating AI technology with existing risk management frameworks. These challenges can vary based on the size of an organization, the amount of data it handles, and the sensitivity level of the data. Regardless, unless these obstacles are mitigated, then harnessing AI in terms of cybersecurity risk management becomes a double-edged sword in terms of regulatory compliance and viability.
Understanding Context
AI algorithms can sometimes encounter issues in terms of understanding context, including evaluating emerging threats. This limitation can be heightened further if the computational resources needed to analyze huge amounts of data are unavailable. This could lead to vulnerabilities being missed and possible false alarms that can lead to wasted time and money. To combat this, AI algorithms need to be designed to have more contextual awareness and trained on as many data sources as possible to reduce any inaccuracies.
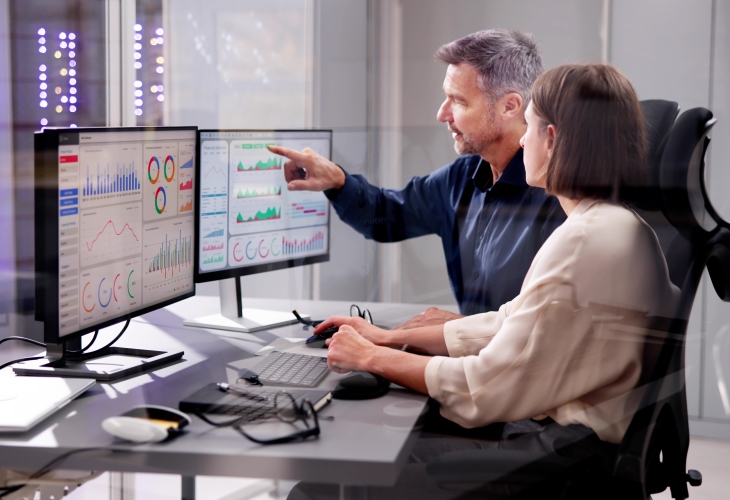
AI Security Systems are Complex
AI-powered security systems can be extremely complex, requiring skilled cybersecurity professionals to configure them and provide ongoing maintenance. Fortunately, more and more tools are reaching the market to make these systems more user-friendly. However, bringing in cybersecurity experts or training existing IT staff can come at a significant cost.
A Lack of Transparency
This complexity can also result in a lack of transparency that makes them difficult to manage, especially for people who are not experts in the field. This means integrating an AI-powered security system may not be the ideal solution without an in-house, highly-paid expert. To help make AI security systems more accessible, the user interface should contain extensive visualizations, analytics, and reporting features to provide clear insights into the software’s decision-making processes.
Cybersecurity Risk Management Software Needs to Be Scalable
AI-powered cybersecurity risk management software needs to be able to grow with the company. This means that algorithms and associated systems and tools need to be scalable, analyzing for new threats and adjusting to increased loads, as and when needed. If the system is not scalable, then it may be quickly left behind in the frenetic world of cybersecurity.
Training Data May Contain Biases
When training any AI or ML model, there is always a chance that the sample data may contain inherent biases that may go unnoticed. This can impact the performance of AI systems and result in costly inaccuracies. To overcome this challenge, AI models must be built using a wide range of data to provide sufficient diversity to avoid underlying bias. Ongoing monitoring is also required to identify any bias with actions taken to adjust and reconfigure the system.
Success Depends on Data
Furthermore, the overall success of an AI system hinges on the quality of the training data. It is crucial not only to train models on diverse datasets but also to ensure the high quality of this data. As with checking for bias, efforts should be made to conduct real-time monitoring and schedule automated checks to remove inaccurate, inconsistent, or irrelevant data. Constantly correcting any errors that are found.
Balancing AI with Human Intervention
Although AI-powered security systems provide a high level of automation, there still needs to be human intervention to ensure everything is working as it should and the system is error-free. AI and human employees should work in collaboration, creating a well-rounded and responsive cybersecurity strategy that is constantly evolving.
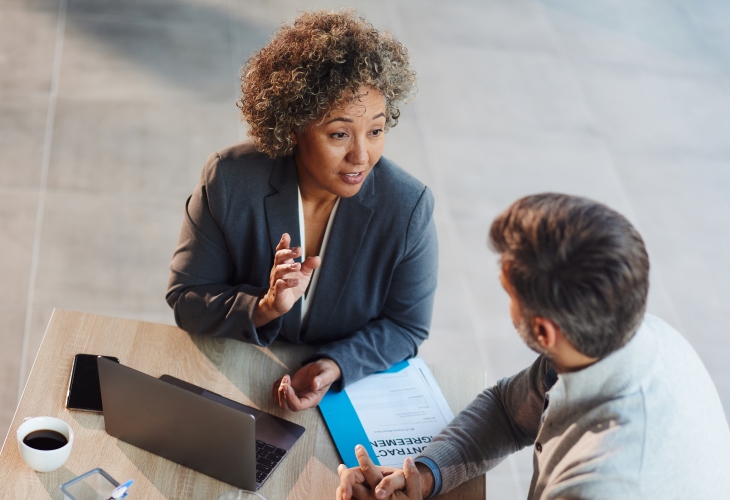
Compliance Issues
Regulations and legal requirements surrounding AI and cybersecurity systems remain unclear and often complicated—compliance guidelines are ever-changing, requiring organizations to constantly stay up-to-date on the legalities of using AI and ML to deliver their security needs. Fortunately, AI is already being used heavily in finance, providing safe transactions, real-time investments, and even assessing the level of risk in cyber insurance. This widespread adoption in such a key industry will likely result in more established regulations that can transfer to other industries, such as IT and cybersecurity.
Ethics
AI is still subject to some ethical concerns, especially where people’s personal information is concerned. When designing risk management software with builtin AI, it is essential to adhere to any ethical guidelines and conduct auditing to ensure the system maintains integrity. This problem is accentuated by the use of AI in other areas of the business, creating a complex web. From budgeting and projections to key transitions like workday staff augmentation, or even hardware maintenance— the more AI is used, the more its ethical impacts need to be considered.
Conclusion
AI will be a mainstay in the future of cybersecurity. Particularly in the development of enhanced cybersecurity risk management. By automating tasks and reducing human intervention, accuracy can be significantly increased. However, AI also presents many challenges that relate to the complexity of systems, ethical concerns, the quality of training data, and so on. This means that AI-powered security systems may not yet be viable for every organization, but the technology is developing rapidly. Therefore, in the coming years, cybersecurity platforms that do not utilize AI will likely become obsolete.