Communication service providers (CSPs) are modernizing their networks to deliver enhanced services to their customers, monetize networks by better serving vertical industries, and, most importantly, improve the performance and cost efficiency of networks. Cloud-native architectures and operational models serve as the foundation of this platform vision.
According to the latest industry insights report from Analysys Mason on Telco Cloud Infrastructure, by 2024, telco network cloud deployments replace the silo network infrastructure deployments, which proves a definite inflection point in how to deploy telecom services on the cloud.
However, as CSPs scale telco cloud deployments, they face daunting challenges to address complexity, manageability, co-existence of new and legacy platforms, automation, and operations of these multicloud platforms, which are fully distributed and deployed at a telco scale.
Telco Networks Transformation and Operational Changes
CSPs are undergoing a radical transformation to reap business benefits. However, a transition from today to future networks is not a Boolean switch as they need to offer multi-generations and multiple hybrid architectures, therefore, building a platform that supports services of today and, at the same time, enables future-proof architecture is not simply good but rather a must-have requirement.
Below are evident challenges in the telco transformation journey. Building the right architecture starts with clear requirements quantification.
The right Artificial Intelligence for IT Operations (AIOps) solution for CSPs must align with unique telco-specific requirements on:
- Use case intelligence – Telco networks have been purpose-built and deployed to deliver critical and distinctive services for decades. These networks are not like data centers but a fleet of resources—such as home networking, fiber, transport, radio, core, cloud, and WAN services. Topology alignment (like A/B plane, Ring) and service resilience are key requirements.
- Management and operations – As network cloud services scale, CSPs face a unique challenge in managing all those instances at a large scale. As an example, a typical T1 CSP can mean 100k+ cloud sites, 10k+ edge sites, and hundreds of regional or central cloud sites. In such scenarios, managing and orchestrating the whole network as one becomes crucial.
- Automation – CSPs are working alongside many standards bodies (especially the TM Forum®) to accelerate automation towards Level-4 (full-service orchestration and automation) and Level-5 (AI-driven automation). However, there still lacks a clear path to apply radical automation for large-scale telco networks.
- Data-driven network – CSP networks, by nature, are highly protected—with network data existing in many silos, building a data-driven network is a complexity that requires a cloud-native operational model and real-time telemetry.
- Intelligent operations – Telecom services need to comply with strict SLAs and regulatory frameworks, resulting in network operations that follow a hierarchical, process-based delivery defined by Network Operations Centers (NOCs). In addition, data knowledge is based on tools and systems that vendors provide. Knowing how to operate networks with modern practices like DevOps and AIOps while at the same time adhering to telecom standards, such as TOGAF® or TMF®, is still an issue to be solved.
Similarly, as operations scale, the risk of human error supersedes the advantages of the cloud.
- Security – Lastly, as networks become open, security concerns become critical. In the modern cloud world, security no longer remains an intrinsic product feature but rather becomes an architecture discussion towards Zero Trust Networks (ZTN).
This is why Communication Service Providers (CSPs), alongside other industries, are looking towards Artificial Intelligence (AI) as an enabler to address the above challenges that can accelerate networks’ autonomous journey.
It is proven by the latest industry insights report published by TM Forum®, which shows CSPs aim to achieve Level-3 automation (conditional autonomous networks) and Level-4 automation (highly autonomous networks) by 2025. There is an increased interest in accelerating Level-5 automation (AI-driven automation) by using AI adoption.
However, telco adoption of AI-centric solutions is not straightforward because most CSPs operate a geographically distributed brownfield network and manage a multi-generation fleet of infrastructure and resources. CSPs also operate at different scales, which means there is no simple, “cookie-cutter” approach towards AI-driven operations (AIOps).
In addition, CSPs adopt solutions based on clearly defined, standard telecom architectures such as ETSI® (European Telecommunications Standards Institute), TM Forum® (Telecom Management Forum), 3GPP® (3rd Generation Partnership Project), and O-RAN (Open RAN alliance). CSPs also source solutions that can interwork and interoperate at a global scale. Finally, CSPs expect these solutions to fully integrate into their brownfield environments.
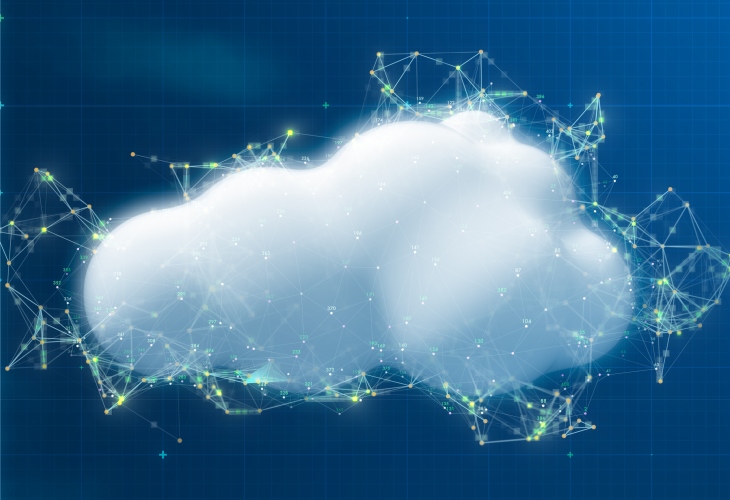
Telco AI Transformation Blueprints
To be successful with AI, CSPs should build a pragmatic AI blueprint for networks, which is outcome-based and supports a phased deployment approach. Below are different transition paths CSPs can adopt to implement AI in a networking environment.
AI on Telco Networks (AoT)
AI on networks delivers a quick business outcome from Machine Learning (ML) and Artificial Intelligence (AI). Already, Operations Support Systems (OSS) and Business Support Systems (BSS) are integrating network data pipelines in existing solutions. Enhancing those systems and consolidating using AI will not only make network architecture flat but also add exponential incremental value.
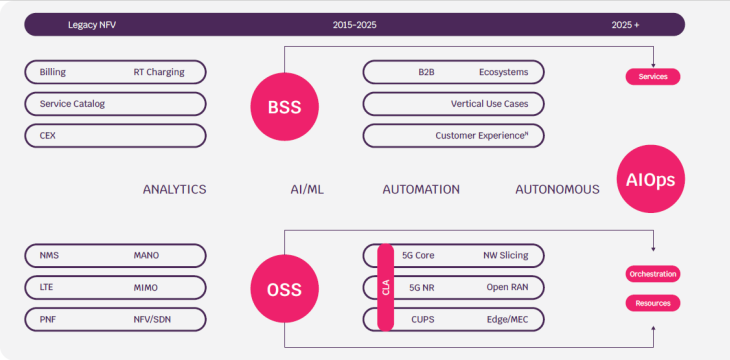
- OSS AI Use Cases – OSS collects feeds from Network Equipment (NEs) and serves as telco operational systems. Integrating AI into them can support:
- Fault Resolution: Root cause analysis, cross-layer co-relation, etc.
- Real-time Alerts: Typically, networks face challenges where dashboarding is not done in real time, then the minimum granularity in most telco OSS systems is not less than 15 minutes, which means intelligent decisions, both from the network availability and the business side, cannot be optimally planned.
- Consolidation: Typically, OSS networks are vertical silos meaning each NFV (Network Function Vendor) brings its own solution making it both complex and inefficient, adopting central OSS with AI promises to evolve for “N” to 1 common visibility layer.
- BSS AI Use Cases – BSS delivers all business and frontend Customer-Facing Systems (CFS). When it comes to customer value, they function as beachheads to deliver value. AI has proven to deliver value in this domain by:
- Virtual Assistants – Virtual assistants and chatbots serve as the most desirable use case to evolve a future BSS architecture.
- Automated Business Packages – Auto recommender systems that can enable the best package proposals to customers, and at the same time, give CSPs real-time policy to monetize their platforms.
- Predictive Complaint Handling – Analyzing customer-specific QoS (Quality of Service) and business metrics to predict issues before they arise.
OSS/BSS are also telecom systems that can best enable CSPs to monetize value from Gen AI (Generative AI), enabling network management and troubleshooting as simple as asking a question “Help me solve internet connectivity for user XYZ.”
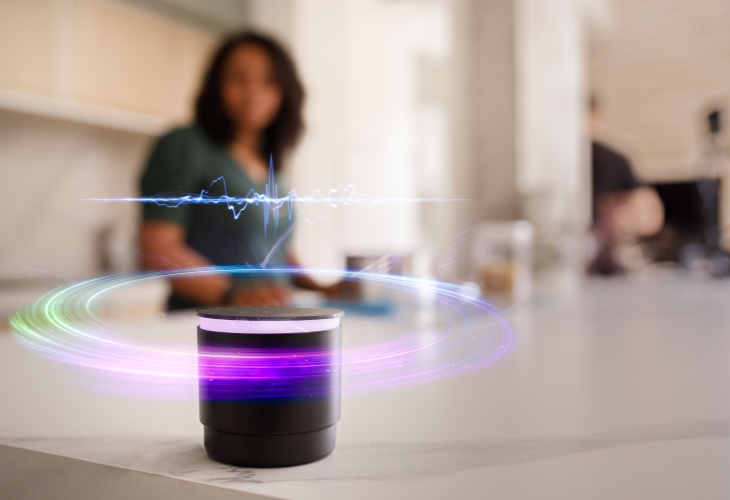
AI by Telco Networks (AbT)
AI by networks deliver a quick business outcome for greenfield deployments. A classic example is CSPs adopting O-RAN (Open RAN) architectures and achieving unique use cases on both automation and efficiency using RIC (RAN Intelligent Controller)
Depending on use cases and location, RIC can be RT (realtime) using AI apps known as xApps or NRT (non-real-time) using AI apps known as rApps that play an instrumental role in enabling intelligent automation and efficiency improvement.
- Network Optimization – AI algorithms are used to optimize network performance, including dynamic resource allocation, predictive maintenance, and load balancing.
- Spectrum Efficiency – AI’s role in enhancing spectrum efficiency is crucial in an era of exploding data demand and limited spectrum resources.
- Anomaly Detection – AI helps in predicting network faults and detecting anomalies, thus, reducing downtime and improving user experience.
- Energy Efficiency – AI’s contribution to making RAN operations more energy-efficient, is a growing concern for operators due to cost and environmental impact.
- Enhanced Quality – AI contributes to personalized user experiences through better management of network resources, QoS (Quality of Service), and QoE (Quality of Experience) improvements.
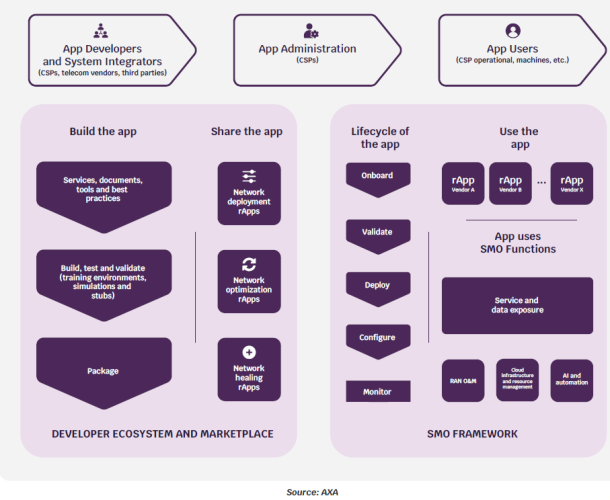
AI in Telco Networks (AiT)
AI in networks delivers a good promise for the early adopter, a key target of AiT is enhancing current solutions with AI add-ons, adding analytics features in network management systems, or delivering smart UE (User Equipment) location tracking are typical examples of AiT.
In Policy and Charging Rules Function (PCRF), AI algorithms are utilized to automate and optimize policy decision-making processes. By analyzing large volumes of real-time data, AI enables more dynamic and efficient management of network resources. This involves the intelligent allocation of bandwidth and other resources based on user profiles, service demands, and network conditions, ensuring optimal performance and adherence to policy agreements.
In the 5G Core, AI plays a crucial role in network slicing, a key feature of 5G architecture. AI-driven systems can predictively manage and allocate network resources for different slices, optimizing each for specific use cases like IoT, high-speed broadband, or low-latency services. This predictive management is critical in maintaining the performance and efficiency of each network slice, particularly under varying load conditions.
Hence, AiT is focused on optimizing operational efficiency and resource management and ensuring high service quality and reliability. These solutions are crucial for meeting the increasing demands on modern telecommunications networks, particularly as they evolve towards more complex and diverse service offerings.
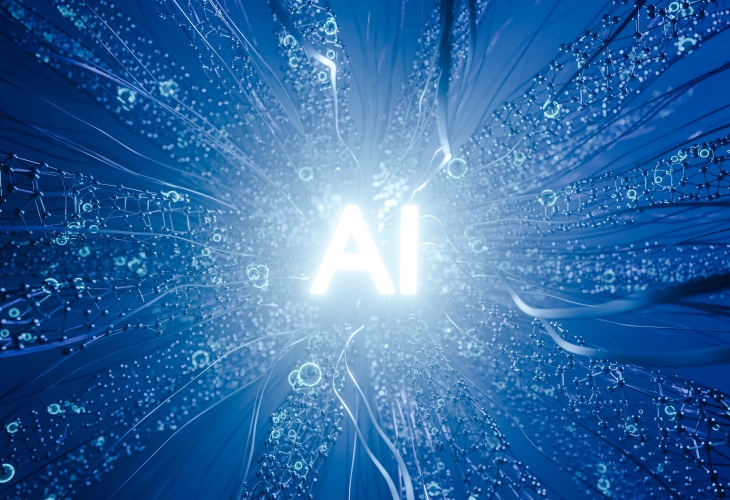
AI on Edge (AiE)
Edge AI in telecommunications represents a transformative convergence of two innovative technologies: Edge Computing and Artificial Intelligence (AI). This integration is particularly significant in the era of 5G and beyond, where the demand for highspeed, low-latency, and data-intensive services is rapidly increasing.
AI “on” Edge has excellent value for CSPs looking to monetize network value from data. It enables use cases based on real-time data insights.
- Edge Computing in Telecom – Edge computing involves processing data closer to where it is generated rather than relying on a centralized cloudbased system. In telecom, this means deploying computational resources at network edges – like cell towers, base stations, or even customer premises. The advantage is twofold: reduced latency, as data does not have to travel far, and decreased bandwidth usage, as only processed, relevant data is sent to the cloud or core network.
- AI at Edge – Integrating AI into this edge framework exponentially amplifies its benefits. Edge AI involves running AI algorithms locally, on the edge devices themselves. This setup allows for real-time data processing, crucial for applications requiring instantaneous decision-making, such as autonomous vehicles, smart cities, IoT devices, and personalized user experiences.
AI on Cloud (AoC)
AI hosted on the cloud can provide significant value to Communication Service Providers (CSPs), expanding their offerings to enterprises. This includes not only connectivity and cloud services but also AI capabilities, which accelerate the adoption of AI ecosystems and use cases.
AI use in networks has gained a lot of attention and is supported by government and international bodies such as the UN WEF (World Economic Forum), however, AI data centers are not only expensive to build but also complex to scale and maintain. This is where validated and ready AI platforms can help CSPs catch the early wave of AI adoption by offering a trusted AI platform.
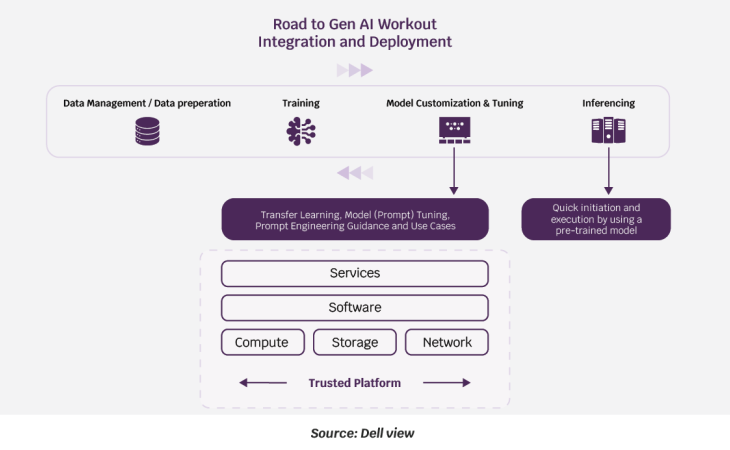
AI API as a Service (AaaS)
AI API as a service refers to the programmability and modularity of AI platforms. To develop AI use cases efficiently, all those resources must be available as APIs and SDKs for the developers.
The use of AI APIs is particularly beneficial in scenarios where businesses seek to enhance their offerings with AIdriven features, such as personalized recommendations, intelligent chatbots, or advanced data analytics. For example, a retail website can integrate an AI-powered recommendation engine through an API, improving customer experience by offering tailored product suggestions based on user behavior.
Similarly, a customer support system can integrate a natural language processing API to interpret and respond to customer inquiries automatically.
AI APIs also facilitate seamless integration and interoperability between different systems and applications. A company can integrate various AI functionalities from different providers into its IT ecosystem, creating a more robust and versatile solution. This integration is typically straightforward, requiring minimal changes to the existing infrastructure.
There is still a need to standardize AI API for networks and adopting an API framework like GSMA® Open Gateway for AI will enable CSPs to both adopt and scale AI at a telco scale.
Data Management and Security for AI
Like other domains, the use of data-driven architectures like data mesh/data fabric for data management and scalability is a necessary requirement for ML/AI. CSP networks represent an invaluable treasure trove of data that can enable smarter networks by enabling telecomready and contextual automation solutions that can simplify operations through predictive use cases like future faults, capacity planning, and resolving network issues before they adversely impact the network. These solutions, over time, will empower CSPs to deliver differentiated outcomes, including pattern recognition and anomaly detection, leading to actionable insights.
AI systems rely on access to great data. Getting your data house in order is a necessary first step to unlocking your organization’s potential with AI. Having access to an Open Modern Data Lakehouse that can process Semi- Structured, Unstructured, and Structured data is key. That means providing for any data type no matter where it resides edge, core, or cloud. By utilizing federated queries and enabling data processing in position, we can streamline the integration of disparate data silos. Providing powerful and high-performance engines to accelerate queries for various workloads and helping gather and prepare data for being used in AI Ops and ML pipelines.
The success of AI in CSPs largely depends not only on data but also on ensuring data management and processing are adequately addressed in each step of the data pipeline, this is why an MLOps framework for Telco use cases is of paramount importance, we need to automate the full data pipeline with necessary trust controls to ensure the trustworthiness of these systems, and addressing customer concerns on AI impacts on society autonomy and human control are critical and right data management systems with security controls is the answer.
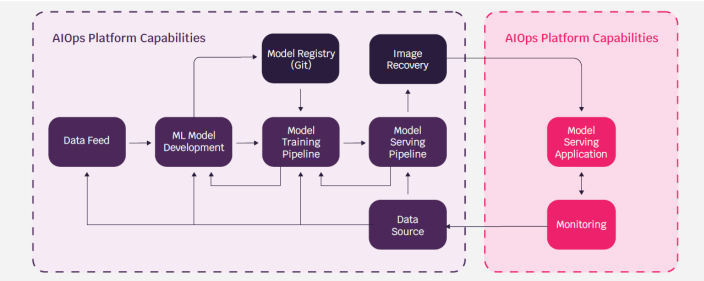
Enabling Intelligent Automation Using AI
Today CSPs are leveraging declarative API and cloud operating models to deploy and manage cloud platforms. The adoption of GitOps and CI/CD models enables us to scale these platforms at scale. However, today, networks have become more complex, and it is mandatory to operate them intelligently. The risk of human errors and security surpass any benefits.
With the adoption of the right automation approaches and data platforms that enable real-time ingestion, CSPs can evolve to Level-5 autonomous networks where AI can automate and adjust network policies based on datadriven insights.
Many CSPs are working to build a Telco-centric LLM (Large Language Model) that is not only tuned based on network data but also aligned with CSPs’ operations process and knowledge base.
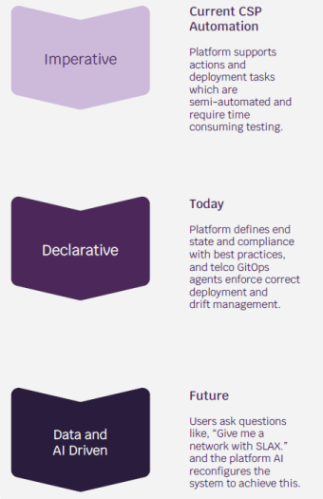
Creating a Secure Trusted Networks with AI
Ushering of cloud and 5G has increased network exposure surface possibility for threat actors to find and exploit network and data, and the possibility with real-time LLMs means hackers have more tools and options to explore. Secondly, as CSPs adopt a data-centric architecture and accelerate automation leveraging AI, there is a continuous risk of trust and hallucination where still more needs to be done towards explainable AI.
According to the latest industry insights, security attacks in the cloud era are increasing an astounding 16% CAGR, and those attacks are more intelligent than before. Defending against those risks in a complex and dynamic environment needs intelligence, and something can only be solved using advanced AI.
Just like in recent years, AI has enabled CSPs to accelerate automation, so are expectations to improve security using AI-driven policy and management. AI brings a new dimension to network security by enabling more advanced and initiative-taking threat detection and response mechanisms. By analyzing vast amounts of network data in real time, AI algorithms can identify and predict unusual patterns or anomalies that might indicate a security breach, such as unauthorized access, malware attacks, or suspicious traffic flows.
This early detection is crucial for preventing potential damage and ensuring network integrity. AI also enhances the adaptability of security measures in telecom networks.
Traditional security protocols might struggle to keep pace with the rapidly evolving nature of cyber threats. In contrast, AI systems can continuously learn from new data, adapting and updating their algorithms to recognize and combat the latest threats. This adaptive security approach ensures that telecom networks remain resilient against both known and emerging threats. Furthermore, AI can automate routine security tasks, such as monitoring, logging, and managing security patches, which reduces the burden on human security teams and minimizes the risk of human error.
This automation not only improves efficiency but also allows security personnel to focus on more complex tasks that require human judgment and expertise.
Trust is another key aspect addressed by AI in telecom networks. By ensuring robust security, AI helps build trust among users and stakeholders, which is essential for the adoption of modern technologies and services, AI-driven security measures can also enhance privacy protection in telecom networks. AI algorithms can detect and mitigate data breaches, ensuring the confidentiality and integrity of user data.
AI is supporting CSPs and enterprises to adopt ZTN (Zero Trust Networks) by delivering unique differentiation.
- Financial Fraud – Fraudulent and illegal use impacts both revenue and reputation, SIM boxes, transit traffic, etc. Using AI and network graphs, all expected traffic flows can be separated from suspicious ones. As networks scale, this becomes paramount to detect and respond to all those threats.
- CVE and Vulnerability – CSP infrastructure teams spend lots of time complying with the latest security standards, NIST, HIPA, etc., but today, scanning practices are a lengthy process that needs to be automated using AI. AI can use code patterns to identify risk.
- Spamming and Behavioral Analytics – AI-powered systems can track user and network activity to spot outliers that might be signs of a security breach. Spam is the biggest threat in social engineered networks where ML/AI has an excellent value.
- Predictive Use Cases – Based on past data and upcoming trends, AI can predict dangers. AI may provide cybersecurity specialists with invaluable information to proactively strengthen defenses by identifying patterns and weaknesses. E.g. 5G network slice assurance ensuring Service Level Agreement (SLA) compliance throughout its lifecycle.
- Automated Response – Using previous data and contemporary trends, AI can predict prospective risks.
- Real-time Authentication – Using AI-driven authentication, users’ and devices’ identities are continually confirmed across all network interactions.
- Access Policies – AI can dynamically modify access rights by ongoing risk evaluations.
- Automated Response – Using previous data and contemporary trends, AI can predict prospective risks.
- Policy and Trust – AI enables dynamic policies that can be adjusted as per network behavior, for example, a trusted device may become un-trusted if it tries to communicate with an unexpected system.
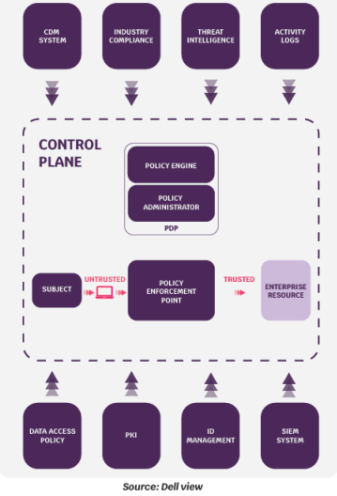
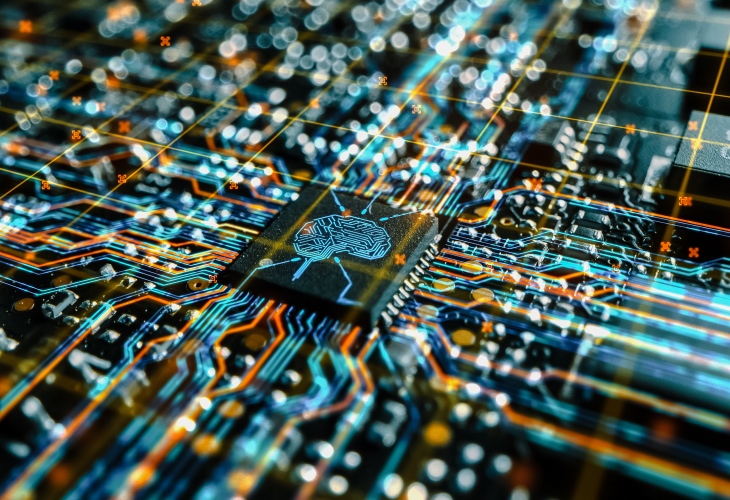
How to Secure and Guardrail the AI Systems
Securing and implementing guardrails for AI systems is paramount to ensure they operate safely, ethically, and effectively.
This process begins with the establishment of robust data governance and ethical guidelines, ensuring AI systems training on high-quality, unbiased data and designed with ethical considerations at their core. It is essential to regularly audit and update these systems to mitigate potential biases that could lead to unfair or discriminatory outcomes. Encryption and secure data practices must be employed to protect the integrity and confidentiality of the data AI systems use and generate.
Another crucial aspect is implementing rigorous testing and validation protocols. AI systems should undergo extensive testing in controlled environments to identify and rectify potential failures or vulnerabilities. Continuous monitoring is also vital to detect and respond to unexpected behaviors or security breaches in real time.
Transparency in AI operations is key to building trust and accountability. This involves clear documentation of AI algorithms, training data, and decision-making processes, making it easier to identify and correct issues. Involving a diverse range of stakeholders in the development and review process can provide different perspectives and expertise, further strengthening the system’s reliability.
Moreover, developing a legal and ethical framework specific to AI operations is critical. This framework should address issues related to privacy, intellectual property, liability, and compliance with existing laws and regulations.
In summary, securing and creating guardrails for AI systems requires a multi-faceted approach encompassing data governance, ethical design, rigorous testing, transparency, and a solid legal framework. This comprehensive strategy ensures AI systems are not only effective and efficient but also operate within secure and ethical boundaries, fostering trust and reliability in these advanced technologies.
Conclusion
ML/AI has great value in a CSP environment, as AI technology continues to evolve, it will enable to build and manage more secure systems. The network’s security posture has transformed by using AI capabilities to learn, adapt, and fix security issues in real time. However, a lot needs to be done to ensure solutions that can inject, process, detect, and act in real time. AI success in the future largely depends on whether we can address data sanity issues and align it with legal/trust frameworks like the EU AI Act, the US Executive order on safe and secure AI, and a number of other local legislations. Industry collaboration to build a successful AI ecosystem is vital for success and to ensure AI delivers on the promise of enhanced security and trust in modern networks.