The COVID-19 pandemic has acted as a catalyst for digital transformation which has gained accelerated attention across enterprises to transform themselves holistically. As transformation becomes quintessential for the survival of enterprises, the journey starts with understanding where the enterprise currently stands and what do they want to achieve from the transformation. The “how” part involves mapping people, processes, and technology to make the journey impactful. It also means ensuring the use of multiple technologies which can be leveraged to meet the goals.
Artificial Intelligence (AI) is recognized as one of the strong technology pillars which enables enterprises to transform themselves digitally. The usage of AI has augmented the human capability in many ways and has enabled enterprises in meeting their business goals. However, the real power of AI can be unleashed if the technology deployment is linked with the overall transformation strategy enabling enterprises to use it in the right places.
Though AI has many use cases, some of the quick wins for an enterprise include projects which involve developing solutions like sales forecasting, predictive maintenance, recommendation engines, etc. As the organization dwells deeper into more complex deep learning-based solution, the ability and performance parameters change dramatically. Deep learning is a subset of AI which can learn from huge amount of unsupervised, unstructured, or unlabeled data. Deep learning techniques are used in most of the Computer Vision (CV) based solutions.
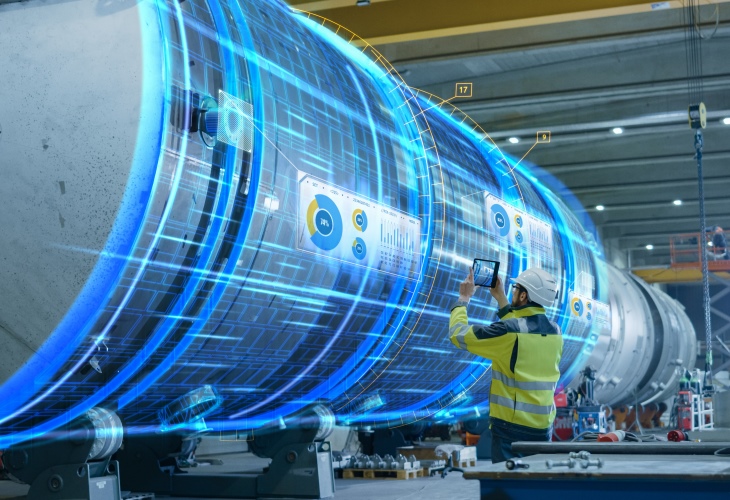
Computer Vision is defined as the ability of the computer to process and identify videos, images, and objects which is deciphered to understand the content and context of a video or image. The content could be anything from an animal, place, object, or human face enabling an enterprise to generate many use cases.
In simple terms, CV is executed through the powerful deep learning algorithm of Neural Networks (NN). NN are a type of interconnected networks which process information like a human brain through a series of connected network elements. NN differentiate and learn by example, hence, they need a lot of training data which gets fed into the computer in the form of raw jpeg images, live camera feed, etc. As data gets fed into the computer, the algorithm learns and deciphers patterns for the multiple data sets and shows output based on its learning. While most of the data ingested in the CV systems are labelled data ingested in supervised manner, modern day CV solutions can also ingest unsupervised and semi-structured datasets.
The rapid development of the CV technology can be attributed primarily to the data in digital format but it is also the low cost of computing power and availability of open-source libraries from organizations like opencv.org which has further augmented the development and adoption of this technology in mainstream industries. AI-assisted computer vision platforms are also capable of functioning in increasingly complex environments. They work continuously and in conjunction with humans, leading to improved efficiency, fewer errors, and better output.
The use of CV is increasingly proliferating across many industries bringing in efficiencies to many business processes and cutting down the cost. Few such industry specific use cases are the following:
Autonomous Cars
Driverless or autonomous cars are now being tested and used with limited mobility space (airport transfers, luxury resorts, etc.). The development modality of driverless cars is that it uses multiple cameras, lidar, radar, and ultrasonic sensors to acquire images from the surroundings so that their self-driving cars can detect objects, lane markings, signs, and traffic signals to safely drive around the area. All of this is a reality, possible through CV, with many manufacturers such as Tesla, BMW, Volvo, and Audi getting deep into developing and commercializing them.
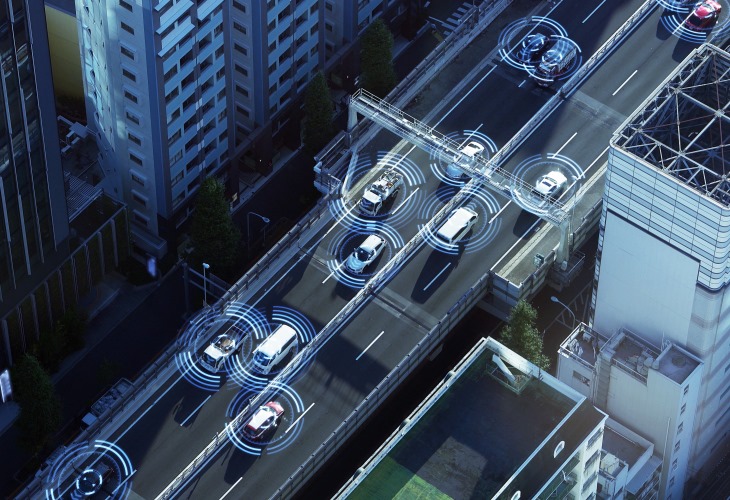
Retail
Retailers have started developing autonomous retail stores which are equipped with overhead cameras. Once the consumer enters the retail stores, it switches its app on. As the customer picks up products, it gets added to his cart and final payment is then made as the customer leaves the premises from his mobile wallet. Such implementation is done by Amazon Go.
Agriculture
A lot of countries are now mapping their agriculture land images captured by satellite and drones. The image data is captured during different times after the seeds are sown which helps in estimating the production quantity of the crop ensuring cost management of future contracts. This information also empowers the government to take preemptive measures beforehand on shortfall or higher growth predictions.
CV in agriculture is also being used to analyze crop quality as it gets harvested and to find the optimal route through the crops, driven by autonomous tractors. John Deere, an agriculture equipment manufacturer, has developed such tractors. A startup in India, Farmbeats, uses drones to map the farm and monitor various crop and soil parameters through the images collected on a regular basis. It is expected in the future that CV identifies weeds so that herbicides can be sprayed on them directly instead of on the crops.
Healthcare
CV provides a plethora of use cases in the healthcare industry. Since 90% of all medical data is image-based, CV-based technology is making quick inroads. From enabling new medical diagnostic methods to analyze X-rays, mammography, and other scans to monitoring patients to identify problems earlier and assist with surgery, CV is playing a very important role in the development of next generation healthcare systems. Tampa General Hospital in Florida deployed a CV-based solution to capture potential patients with symptoms of COVID-19.
Manufacturing
CV is also helping manufacturers to run more safely, intelligently, and effectively in a variety of ways. It is not only helping them to monitor the equipment and raise an alarm before the breakdown happens. It is also being used for packaging and product quality monitoring by identifying defective products.
Logistics
Logistics providers like DHL and Amazon are using CV in their warehouses to sort different products which is helping them to improve the efficiency by reducing the sorting time.
Banking and Financial Services (BFSI)
BFSI is changing the way they work and slowly adopting CV in their business processes with different use cases. From retail to commercial banking and insurance, it has different use cases. It is being used to bring in efficiencies in processes concerning fraud detection, enhancing cybersecurity enhancing customer experience, back and front office processing, etc. CaxiaBank is a Spain-based bank which allows its customers to use facial recognition technology to draw cash from its ATMs — improving user experience and enhancing security. The claim processing in the insurance industry is still a tedious task, however, the use of CV in claims processing has started to make the work of insurers somewhat easy. China Pacific Insurance (CPIC) has transformed its claim processing by using CV-based solutions in partnership with Baidu which has tremendous impact on the operational efficiency.
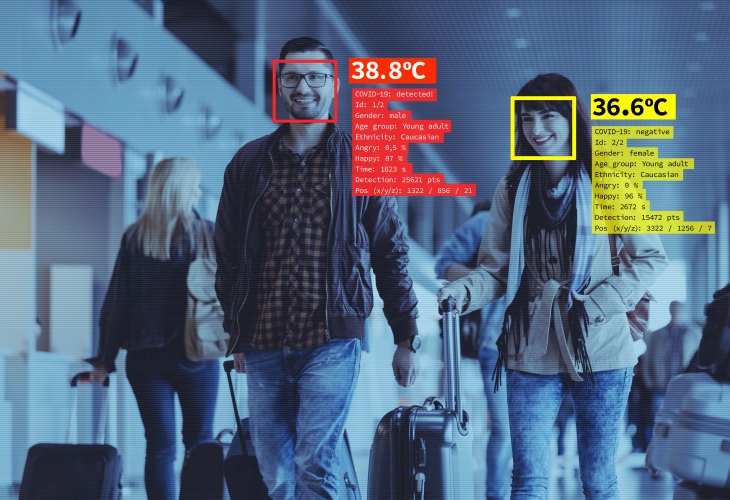
Security
CV is also being used to augment the security of strategic locations. The facial recognition solution running at the backend constantly scans for unidentified people and raises an alarm on finding one. China is much ahead in using facial recognition technology, and they use it for police work, payment portals, and security checkpoints at the airport. The facial and vehicular recognition solution deployed at Taoyuan International Airport in Taiwan helps authorities to boost the safety of the airport and shorten response times in case of any emergency.
However, there are many challenges in developing a CV-based solution:
Huge data requirement: Solutions like facial recognition or autonomous cars require huge data sets to train the algorithm. It is certainly difficult for machines to process all the image and video data when training a computer vision model. While today there are no computational problems due to the availability of GPT-2 & GPT-3, doing a CV solution based on live video feeds is still a complex task.
Privacy threat: A lot of CV-based solution encroach upon the privacy of individuals and cannot be implemented due to various regulatory and complaint issues like GDPR, PDPA, etc. Considering privacy threats, governments of a lot of countries have either banned facial recognition-based solution or ensure strict regulatory adherence.
As much as CV is constantly evolving into a better technology and solving various use cases, it is difficult to simulate the actual vision of human into a machine. Such marvel will take few years before machines can perfect it. As AI is still perfect at doing one task at a time, doing complex work through CV will take some time for perfection and becoming into a fully mature technology.