In 2023, data has exploded. Across the globe, corporations are handling ever-increasing amounts, which has proved to be a double-edged sword.
Inarguably, this has led to better service provision and linking between organizations, so that customers benefit from a more joined-up world.
On the downside, the proliferation of data has led to a growing risk of cybersecurity breaches. In the same way that an unwanted consequence of having more bank branches is more bank robberies, the more data there is flying around, the more likely it is to be stolen.
The result is that cybersecurity is a huge issue, with both internal and external organizations offering ways to enhance the data safety of a business. One of these is to use data analytics.
We take a look at what this means for your organization in this article.
Why is Cybersecurity an Issue?
Put simply, cyber threats are an issue for both companies and customers. For the former, there are often huge fines for security breaches, with the average fine for data leaks in the US set to rise above $5 million this year.
As far as GDPR breaches go, the fine can be as much as two percent of the global turnover of the company. This can cripple an organization—and not just financially. The hit to the company’s reputation can also be hard to recover from.
Apart from all that, cybersecurity is the moral duty of any business charged with looking after confidential data. Organizations involved in this activity should take the responsibility profoundly seriously, as should all individuals within these companies, whether you hire cybersecurity freelancers or depend on an in-house team for your data protection needs.
One of the ways this responsibility can be discharged with the greatest effectiveness is through data analytics. If you look at the graph below, you can see that data breaches and their impacts have been on an upward trend in the US for many years now.
With the growth in IoT and cloud computing spelling the emergence of a whole lot of new vulnerabilities that require monitoring, the need for data analytics has never been so pressing.
So, whether you are looking to strengthen your email security or want to partner with one of the top cloud providers without inadvertently creating new weaknesses or entry points, here’s what you need to know.
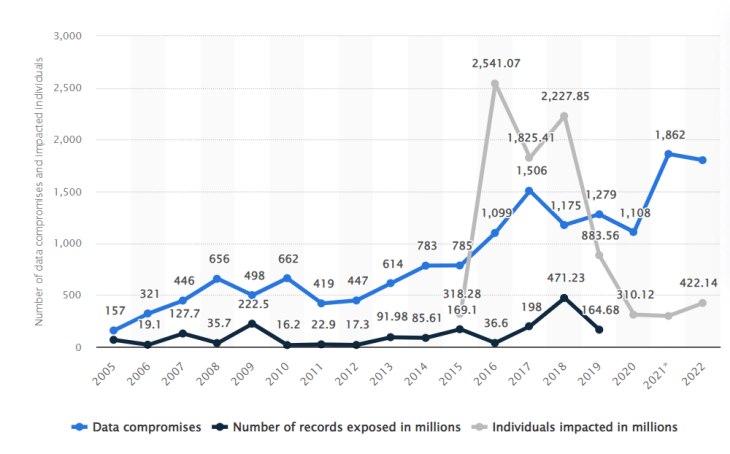
What Does Data Analytics Entail?
Until quite recently, when seeking to answer the question “What is a cybersecurity risk assessment”, big data organizations would typically have followed a; prevent, detect, respond (PDR) strategy.
This was the traditional method of adopting fundamental steps for security (for example, having staff change their passwords every month), keeping an eye out for dangers, and putting responses in place in the network infrastructure to counter these. For a time, this piecemeal approach worked alright.
However, with the recent growth in cybersecurity threats, new approaches and a better security infrastructure have been called for. This is where data analytics is so useful.
But what does this look like? There are several steps involved in getting it right, which we will consider below.
1. Data Collection
The first action a data analyst will take when confronted with a cybersecurity environment is to conduct a thorough accumulation of key data, such as authentication, web activity, and network information. It is important to be as wide-ranging as possible to construct a complete picture of the routes data takes and where its vulnerabilities may lie.
Any activity logs that contain this kind of information constitute an attack vector for a cybercriminal, so an additional step at this stage is to move these logs somewhere with enhanced protection.
2. Data Classifying
A lot of the information gathered during this first step will be somewhat raw and unstructured i.e. the kind of data commonly encountered in a lakehouse platform. For its utility to be optimized, it needs to be categorized. By using field names such as port, user, timestamp, etc., this data becomes more usable. Subsequent meaningful analyses can now be run. Searches of this data become easier and quicker, and threat vigilance routines can be run with greater speed.
3. Expansion
Now the analyst has a framework for their data, it befalls them to go back to the collection stage to see what they have missed. Any residual information can be inserted into the taxonomy they have established. Obviously, over time, more data is generated, so this step is all about keeping analytics current.
This underlines how complete the data picture you are trying to construct has to be.
4. Enrichment
At this stage, information from other sources, such as threat vigilance feeds and machine data, is fed into the framework. In this way, the analyst gains a good idea of the latest threats circulating to go with their understanding of the strengths and weaknesses of the organization’s data security.
It is similar to a shark expert constructing a cage based not just on the properties of the alloy being used but also on the kind of shark threat they are likely to encounter in that part of the ocean.
The idea is to put in place 360° visibility so potential threats are picked up early and the whole cybersecurity team can swing into action when alerted.
As well as this, the security analyst needs to construct a system that records the details and context of the threats in order for improvements to be made in the wake of attacks. Every incursion is an opportunity to learn, and this is one of the fundamental roles of data analytics.
5. Automation
In the joined-up world where businesses operate 24/7 and data flow never stops, it is clear that threat detection has to be automated. There is no way individuals can be tasked with such a non-stop responsibility, and to share the activity among a sufficient number of people would be a very large staffing investment.
Therefore, automated systems must be put in place, which can detect likely and not-so-likely threats, triage their severity, apply solutions, and update all stakeholders. There will need to be an established threshold, too, at which point human actors have to sign off. Apart from that, it all has to work without a hand on the tiller.
One of the big advantages of automation is that it delivers data in real-time. In other words, the analyst does not have to stop the cybersecurity system in order to derive insights. Automation gives a constant feed while the system continues to function unimpeded.
6. Detection with Machine Learning
Once the system is up and running and threats are being scanned for, the operation will grow ever more vigilant and attuned because of machine learning methods—one of the hallmarks of artificial intelligence.
The eventual ambition is for the system to be watertight against unknown unknowns, which is a big ask. However, with the use of a data analytical perspective that is as wide as possible, the system can learn what the threat landscape contains and extrapolate therefrom to include novel dangers. In this way, more effective detection of threats is rendered.
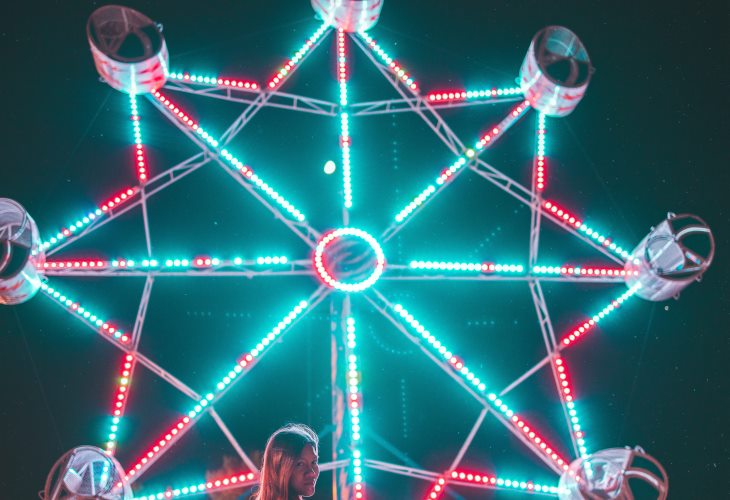
What Can Data Analytics Contribute to Cybersecurity?
- Security Orchestration, Automation, and Response (SOAR)
This is a data analytics approach that emphasizes the importance of the process junctures between data gathering, analysis, and threat response. It requires the analyst to think holistically, incorporating information wherever there is common access, whether it be clean room data or another shared form of information.
In an increasingly connected world, the joins are where some of the most telling insights can be found.
- Threat Intelligence Software
This is a proactive approach that gives an up-to-date picture of what is happening in the world of cybersecurity i.e. the latest threats and the newest ways of dealing with them.
- Forensics
This is similar to when the CSI team comes in to analyze exactly what happened while the evidence is still fresh. When a security incident has occurred, data analytics can be used to assess how weaknesses in the operation were exploited and give actionable insights to help remedy these shortcomings.
- Predictive Analytics
This is a statistical means of establishing threat likelihoods. It not only delivers verdicts on external threats but also where special vulnerabilities are.
A classic example of the latter is when a user group that has enhanced authorization on a system is not required to apply enough rigor to its access credentials. They may be allowed to use the dreaded ‘password’, for instance.
Heat maps and chronological timelines can be generated and used to analyze exactly where the biggest stress points in an organization are.
- Network Analysis and Visibility (NAV)
This form of data analytics is concerned with data flow. It looks at traffic levels and tries to identify pinch points to ease flow and improve security.
- Malware Analysis
This is where data analytics gets to grips with malicious software by isolating any instances in a safe environment and then applying the principles of data analytics to find out as much as possible about the malware’s attack mode. This is then added to the machine learning system so greater readiness can be developed in the fight against malware attacks in the future.
Staying Cybersecure with Data Analytics
Unfortunately, as the saying goes, the first casualty of war is truth. But, in a hostile environment, accurate information is everything. Consequently, if your business is going to survive against a legion of threats, it needs to work hard to get a clear picture of what is occurring.
This is the core strength of data analytics. It seeks, through formalized steps, to give a body of data that is accurate and highly usable in the fight against cyber incursion.
In short, data analytics tells you what is going wrong and where it is going wrong, offers solutions, and institutes the means for continuous improvement. Can you risk being without this in your cybersecurity strategy?