Artificial Intelligence (AI) is rapidly transforming how we interact with digital technologies, pushing the boundaries of what machines can do and how they can learn. As AI continues to evolve, it increasingly becomes a double-edged sword: on one side, it drives innovation and efficiency, and on the other, it introduces new complex cybersecurity vulnerabilities that can be exploited maliciously.
The Evolution of Cyber Threats with AI
Think of AI as a master key. In the right hands, it unlocks potential and opportunity, enhancing productivity and innovation. In the wrong hands, however, it becomes a tool for criminals, adept at picking through the digital defenses of corporations and individuals alike. This section explores how AI has transformed the landscape of cyber threats and what that means for cybersecurity.
Initially, cyber threats were fairly straightforward and could be mitigated with basic tools like antivirus software and firewalls. These early threats included simple viruses, worms, and basic phishing scams. Over time, as defenses improved, attackers adapted their techniques. Today, however, attackers use sophisticated AI tools to learn from and adapt to security measures, finding ways around traditional defenses with alarming speed and efficiency. For instance, machine leaalgorithms can analyze vast amounts of data to identify patterns and weaknesses in a system. This capability allows attackers to launch more precise and devastating attacks. Unlike traditional methods, AI can continuously improve its strategies, making it a formidable adversary in the cybersecurity landscape.
Current Trends in AI-Powered Cyber-Attacks
The rise of AI has given birth to new forms of cyber threats that are more sophisticated and harder to detect.
Here, we delve into some of the most concerning AI-driven cyber threats.
- Generative AI-Driven Attacks: These involve the use of AI to automatically generate attacks that are increasingly sophisticated and difficult to distinguish from legitimate interactions. For example, deepfake technology, powered by AI, can create realistic audio and video clips of individuals, potentially used to manipulate or compel individuals into divulging confidential information.
Deepfakes can simulate someone’s voice or image with high accuracy, leading to scenarios where attackers can impersonate executives or colleagues, convincing employees to transfer funds or share sensitive data. - Ransomware Innovations: AI-driven ransomware attacks have become increasingly prevalent, where AI algorithms quickly identify the most critical data within a system and encrypt it, maximizing the impact of the attack and the ransom that can be demanded. Traditional ransomware may indiscriminately encrypt all data, but AI-enhanced ransomware can target backups and critical files first, ensuring that victims are more likely to pay the ransom.
- Supply Chain Vulnerabilities: As supply chains become more digitized, they also become more vulnerable. AI can analyze vast amounts of data to identify the weakest link in a supply chain, allowing attackers to initiate a cascading effect that compromises interconnected systems. For instance, a compromised supplier can serve as a gateway to attack larger, more secure companies downstream, creating a domino effect.
- AI-Enabled Espionage: With the ability to process and analyze large datasets quickly, AI has become a powerful tool in cyber espionage, enabling attackers to uncover and extract valuable data without the heavy footprint of traditional hacking methods. AI can sift through emails, documents, and communications at an unprecedented speed, identifying sensitive information and patterns that human hackers might miss.
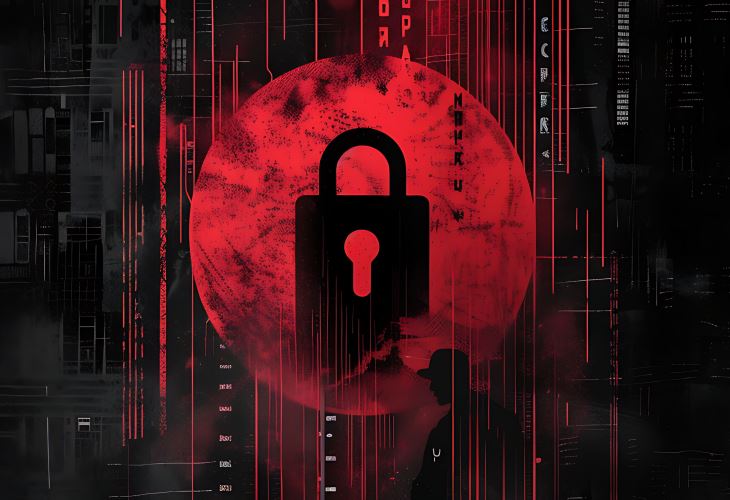
Deep Dive into AI-Driven Security Breaches
This section explores several high-profile AI-driven cyber incidents, detailing how they were orchestrated and the lessons learned. One notable example is the 2020 Twitter hack, where attackers used a combination of social engineering and AI to compromise high-profile accounts. By analyzing the behavior and communication patterns of Twitter employees, attackers crafted highly convincing phishing messages. Once they gained access, they used AI to coordinate a Bitcoin scam, posting messages on compromised accounts and monitoring responses in real-time to maximize their impact. Another example is the attack on a major energy provider in 2021. Attackers used AI to breach the company’s network by identifying and exploiting vulnerabilities in their operational technology (OT) systems.
The AI algorithms used by the attackers were able to navigate the network, evade detection, and ultimately disrupt critical infrastructure operations, causing significant financial and operational damage.
These incidents highlight the growing sophistication of AI-driven cyber-attacks and underscore the need for robust defense mechanisms.
Mitigating AI-Powered Cyber Threats
Battling AI-powered threats requires a multifaceted approach, blending traditional cybersecurity measures with innovative AI-driven solutions.
- Advanced Threat Detection Systems: Utilizing AI to detect and respond to threats is becoming essential. These systems use machine learning to recognize patterns and anomalies that may indicate a security breach. For example, AI-driven systems can analyze network traffic in real-time, identifying unusual patterns that might suggest an intrusion. By learning from past incidents, these systems can improve their detection capabilities over time.
- Robust Encryption Practices: Ensuring that all sensitive information is encrypted effectively can reduce the severity of breaches when they occur. Advanced encryption algorithms make it significantly harder for attackers to access and use stolen data. It is also crucial to implement end-to-end encryption, ensuring data is protected at rest and in transit.
- Regular Security Audits and Updates: Continuous monitoring and updating of security protocols is essential in keeping up with rapidly evolving cyber threats. Regular audits help identify potential vulnerabilities and ensure that security measures are up to date. Patching systems promptly to address known vulnerabilities is critical in preventing exploitation by attackers.
- Employee Training and Awareness: Regular training sessions for employees on the latest cyber threats and best practices is crucial. This includes teaching them how to recognize AI-generated phishing attempts and other deceptive strategies. Awareness programs should emphasize the importance of a cybersecurity hygiene, such as using strong passwords, enabling multi-factor authentication, and being cautious with email attachments and links.
- Implementing Zero Trust Architecture: The zero trust model operates on the principle of “never trust, always verify.” This approach assumes that threats could be both external and internal, and as such, it requires verification at every stage of digital interaction. By segmenting networks and enforcing strict access controls, organizations can limit the potential damage from compromised accounts or devices.
Ethical Considerations and Regulatory Compliance
As AI continues to be an integral part of cybersecurity, ethical considerations and compliance with regulations become increasingly important. This section discusses the ethical use of AI in cybersecurity, focusing on privacy concerns, the potential for bias in AI algorithms, and the importance of transparency in AI-driven security measures.
- Privacy Concerns: AI systems often require access to vast amounts of data to function effectively. This raises significant privacy concerns, especially if sensitive personal or corporate information is involved. Organizations must ensure that data collection and processing comply with privacy regulations, such as GDPR and CCPA.
- Bias in AI Algorithms: AI algorithms are only as good as the data they are trained on. If the training data is biased, the AI system may also exhibit bias, leading to unfair or discriminatory outcomes. It is essential to regularly audit AI systems for bias and take steps to mitigate it, such as using diverse and representative training data and implementing fairness-aware algorithms.
- Transparency and Accountability: Transparency in how AI systems operate and make decisions is crucial for trust. Organizations should strive to explain AI-driven decisions in understandable terms, particularly when these decisions impact individuals. Additionally, there should be mechanisms for accountability, ensuring that there is a way to challenge and review AI-driven outcomes.
Future Directions in Cybersecurity
Looking ahead, the field of cybersecurity is set to become a battleground not just of wits but of AI capabilities. This section speculates on future trends in AI and cybersecurity, including the development of autonomous security robots, the use of blockchain to secure AI transactions, and the potential for quantum computing to revolutionize how we protect digital assets. Autonomous Security Robots: As AI continues to advance, we may see the deployment of autonomous security robots capable of patrolling and monitoring physical and digital spaces.
These robots could use AI to detect and respond to threats in real-time, providing an additional layer of security for critical infrastructure and sensitive environments.
- Blockchain and AI: The integration of blockchain technology with AI has the potential to enhance security in several ways. Blockchain’s immutable ledger can ensure the integrity of AI transactions and data, preventing tampering and unauthorized access. This combination could be particularly useful in securing supply chains and critical systems.
- Quantum Computing: Quantum computing holds the promise of solving complex problems that are currently beyond the reach of classical computers. In the context of cybersecurity, quantum algorithms could potentially break current encryption methods, necessitating the development of quantum-resistant encryption. On the flip side, quantum computing could also be used to create more secure encryption methods and improve threat detection capabilities.
- AI-Driven Cybersecurity Insurance: As cyber threats become more sophisticated, the demand for cybersecurity insurance is likely to grow. Insurers may increasingly use AI to assess risk and determine premiums, offering more personalized and dynamic coverage based on an organization’s specific threat profile and security measures.
Collaboration and Information Sharing
In the fight against AI-powered cyber threats, collaboration and information sharing are crucial. Cybersecurity is a collective effort, and no single organization can tackle these threats alone. This section highlights the importance of sharing threat intelligence and best practices among organizations, industries, and governments.
- Threat Intelligence Sharing: By sharing information about cyber threats and vulnerabilities, organizations can better prepare and defend against potential attacks. Threat intelligence platforms and industry-specific information sharing and analysis centers (ISACs) play a critical role in facilitating this exchange of information.
- Public-Private Partnerships: Governments and private sector organizations need to collaborate closely to address the growing cyber threat landscape. Public-private partnerships can enhance the overall cybersecurity posture by pooling resources, expertise, and intelligence. Joint initiatives and frameworks can help standardize responses and improve resilience.
- Cybersecurity Education and Workforce Development: Investing in cybersecurity education and training is essential to building a skilled workforce capable of defending against AI-powered threats. Organizations should support initiatives that promote cybersecurity awareness and provide opportunities for professionals to upskill and stay current with evolving technologies.
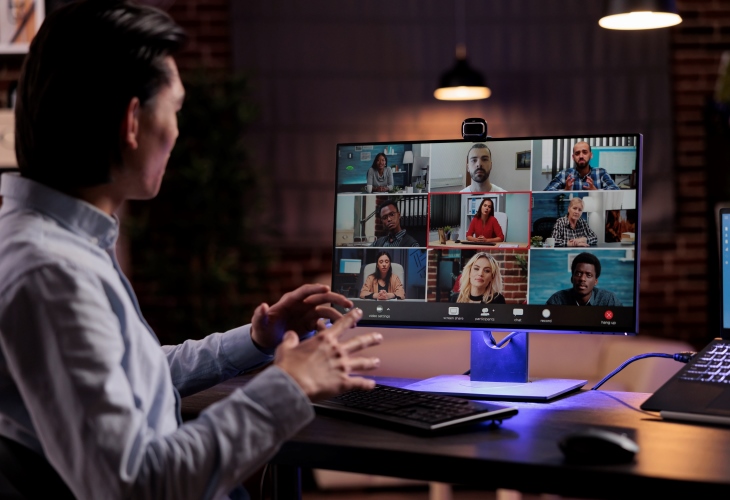
Case Studies and Real-World Applications
To illustrate the practical implications of AI-powered cyber threats and the effectiveness of mitigation strategies, this section presents detailed case studies of real-world incidents and how they were addressed.
- Case Study 1: Financial Sector Breach: In 2022, a major financial institution experienced a sophisticated AI-driven attack that compromised customer data. The attackers used AI to analyze network traffic and identify vulnerabilities in the institution’s security infrastructure. The breach was detected by an AI-driven threat detection system that identified unusual patterns in data access. The institution responded by implementing stronger encryption, enhancing access controls, and conducting extensive employee training on recognizing phishing attempts.
- Case Study 2: Healthcare Data Breach: A large healthcare provider faced an AI-powered ransomware attack in 2021. The attackers used machine learning algorithms to target critical medical records and backups, encrypting them and demanding a ransom for their release. The provider’s response included deploying advanced AI-driven threat detection tools, restoring data from secure offline backups, and collaborating with law enforcement to track and mitigate the attack. The incident underscored the importance of regular backups and robust incident response plans.
- Case Study 3: Government Agency Cyber Espionage: In 2020, a government agency was targeted by an AI-enabled espionage campaign. The attackers used AI to analyze communications and extract sensitive information related to national security. The breach was detected through anomaly detection algorithms that identified unusual data access patterns. In response, the agency implemented stricter access controls, enhanced monitoring of privileged accounts, and conducted a comprehensive review of its cybersecurity policies.
The Human Element in Cybersecurity
While AI and advanced technologies play a crucial role in defending against cyber threats, the human element remains vital. This section discusses the importance of human expertise and judgment in cybersecurity, emphasizing the need for collaboration between humans and machines.
- Human-AI Collaboration: AI can augment human capabilities, but it cannot replace the need for human judgment and decision-making. Cybersecurity professionals bring critical thinking, creativity, and contextual understanding that AI currently lacks. Effective cybersecurity strategies leverage the strengths of both humans and AI, creating a synergistic defense mechanism.
- Continuous Learning and Adaptation: The cybersecurity landscape is constantly evolving, and so must the skills and knowledge of professionals in the field. Continuous learning and adaptation are essential to staying ahead of emerging threats. Organizations should invest in ongoing training and development programs to ensure their cybersecurity teams are equipped to handle the latest challenges.
Conclusion
As AI technologies continue to evolve, so do the methods by which they can be exploited. Understanding the risks associated with AI-powered cyber threats and implementing robust defense mechanisms are crucial for protecting digital assets. By proactively enhancing our defenses, fostering a collaborative security environment, and addressing ethical considerations, we can mitigate the impact of AI-driven cyber-attacks.
In conclusion, the future of cybersecurity lies in a balanced approach that combines advanced technologies with human expertise. By staying informed, continuously adapting, and working together, we can safeguard our digital world from the malicious use of AI.