“The number 1 thing that has made us successful by far is a compulsive obsession with the customer” Jeff Bezos
We are all customers. And we all know what a great customer experience feels like. Be it the comfort of an attentive and friendly maitre’d at your favorite restaurant, the delight of unboxing and turning on a shiny new phone, or having a call center query dealt with seamlessly – in a way that makes you feel like you matter to the company.
Companies such as Amazon, now the world’s largest retailer, have owed their success, by owing to their relentless obsession with ”the customer”. In 2021, it is no longer about just providing a great customer service like Amazon used to do so well. In order to compete, build loyalty, and achieve market dominance now, companies need to ask themselves how they can shape the experience that each customer has with the brand as an individual; how they can be relevant and accessible in the right moments; and how they can predict what the customer wants next. Essentially they need to influence the things that truly affect how the customer experiences the brand and the things that really matter to them, when making a purchasing decision.
“How can companies win the hearts and minds of customers through exceptional experiences?”
AI as a technology applied to improving customer experience presents both a threat – of losing out to competitors or wasting resources through failed implementations, as well as an opportunity to outcompete rivals and delight customers. In this piece, I hope to highlight the breadth of what is possible in customer experience improvement and provide insight into common challenges that I have seen companies face when embarking on this path.
Technical note: for the purposes of this article, I refer to a broad definition of artificial intelligence which encompasses almost all algorithms associated with machine learning and data science, including, but not limited to, linear regression, deep learning, and more. From a business perspective, too much time and effort is wasted on trying to delineate artificial intelligence from adjacent fields such as statistics or vice versa. It is more useful to focus on the business value being created.
How AI is being used to improve customer experience
Customer experience is a customers’ internal and subjective response to any direct, for example through purchase or use, or indirect encounters, such as adverts or branding. It covers every element of a company’s offering, including advertising, purchasing, packaging, product and service features, simplicity of use, and reliability, among others. Therefore, every function of a company has a role to play in creating exceptional customer experiences. Considering this broad definition, there are four ways that I have seen AI used to improve customer experience:
1. Measuring and managing the customer experience
The two most widely used measures, customer satisfaction (CSAT) and Net Promoter Scores (NPS), fail to tell companies what customers really think and feel and can even mask serious problems. NPS and CSAT scores are often taken periodically (perhaps once per quarter) or at the end of an interaction, but they cannot be real-time.
As many customers today use digital, real-time services or apps, firms can increasingly gather more real-time data about customer interactions instead of relying on simplified, single-metric ways of measuring customer experience. By combining these data with insights from direct customer comments (e.g. processed using natural language processing algorithms), analysis of customer transactions and other sources can provide companies with a deep and 360-degree view of the customer experience. For example, one customer could give a company a nine on the NPS score, but in the comments, the customer noted that “Everything was great, but I was disappointed with the check-out process and how long it takes”.
Using AI in this way has several benefits including, capturing emotional responses in real-time, providing deeper analysis to show what you’ve been missing, training your staff by showing them what’s important to customers, and helping you prioritize the actions important to customer experience.
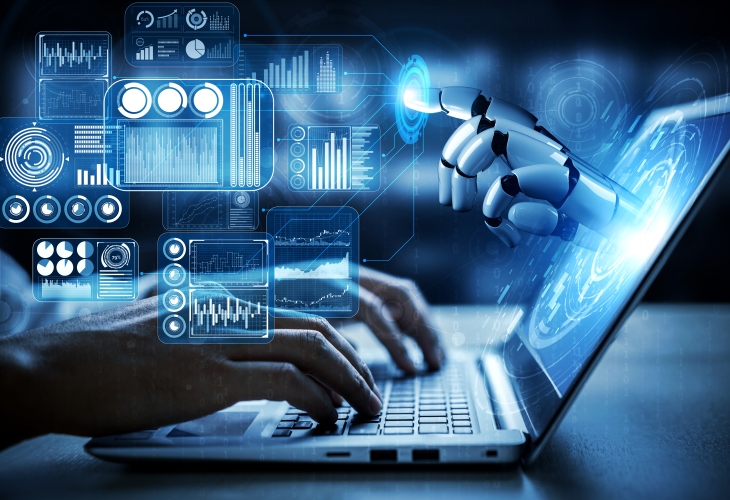
2. Developing AI-enabled products and services
Personalization and contextual awareness
Here AI systems may use customer profile data, location data, browsing history, purchasing decisions, etc., in order to personalize customer experiences and deliver an optimal experience that is appropriate to the context in real-time.
This is targeted to individual customers or small groups of customers. Imagine a hotel that can anticipate what you like and set the room up just right for you before you arrive.
Everything from the type of pillow you like, whether the blinds are drawn or not, whether you like still or sparkling water, and where the temperature of the room could be customized to just the way you like it.
But not just that, it would introduce contextual awareness by taking account of the time of year when setting the room temperature and the location and which way the room faces when setting the blinds.
Empowering self-service
A very common use of AI is the elimination of waiting “onhold” for the next available agent to address a customer complaint (at least when it works well). Many companies now commonly use chatbots and virtual assistants to answer common customer service questions. Innovative Website hosting platform, Wix.com, has developed an ADI (artificial design intelligence) system. Customers feed the ADI information about themselves, the site they want to create, and some personal design preferences, and the site automatically generates a tailor-made site for you, making it quicker and cheaper than using traditional web designers.
Always-on available-everywhere experiences
Automating intelligent systems using AI allows for customers to have 24/7 support and access to platforms. This is something that most companies could not provide at all. Many customers do not want to wait until the start of the business day to contact them. A Stanford-developed algorithm called CheXNeXt is recently able to check chest x-rays for 14 different pathologies simultaneously and more accurately than doctors. Technology like this can one day dramatically improve patient experience in-hospital as it allows for results to be generated much quicker and at any time of the day or night. It is especially useful in remote regions where specialist doctors may not be available.
Smart user-interfaces / interactions
AI may be used to create unique experiences for customers interacting with products and systems. Smart interfaces allow the interface to adapt to the user and the context that they are operating in. Microsoft Word underwent a major interface refresh a couple of years ago, and now the interface is able to adapt not just to the size of the screen but also the context of what the user is doing and the controls they may need next.
3. AI-enabled operations to enhance customer experience
AI applications in back-end operations can dramatically impact customer experience because so many aspects of a business affect customer experience. For example, a colleague of mine was complaining to me recently about how his bank would decline his credit card transaction every single time he tried to purchase air time from a certain cell phone company; and every time he would have to call them to investigate and clear the transaction. He was clearly beside himself with frustration. I happened to be aware that this particular bank had just implemented a new AI fraud detection model – a clear example of how AI and its accuracy and implementation can have a major impact on customer experience. In the insurance industry, many insurance carriers have changed the amount of information that they request in order to provide a client with a quote. In the past getting an insurance quote in many markets involved completing a lengthy questionnaire primarily to feed their quoting engines; today through the use of more advanced data science, extensive data augmentation, and a decision by companies to be more customer-focused, these forms can be as short as just four questions long.
Using AI to design customer experience
AI may be used in innovation and product development for new products. Commonly, different designs may be tested against each other by using processes such as A/B testing. This is a randomized experiment wherein two or more versions of a design are shown to different segments of customers at the same time. Feedback data is collected either through observing results or collecting feedback from customers to determine which one leaves the maximum impact or drives business metrics the most. Using generative design algorithms that rely on machine learning techniques to factor in design constraints and provide an optimized product design. In 2018, General Motors adopted Autodesk generative design software to optimize for weight and other key product criteria for a seat-belt bracket.
The solution was recently tested with the prototyping of a seatbelt bracket part, which resulted in a single-piece design that is 40% lighter and 20% stronger than the original component design.
Common challenges
While the opportunities to apply this technology to improve customer experience are now clear, it is certainly not an easy journey in my experience. AI projects, in my experience, are riskier in themselves owing to the difficulties associated with data, the costs involved, and the inherent uncertainty. Being innovative with AI can therefore be doubly risky. Below I highlight some of the key challenges in my experience.
Innovating with AI
In recognizing that artificial cognition is only very loosely analogous to human cognition and often not at all analogous, it becomes apparent why it can be difficult to imagine what is possible when designing products with AI. We are dealing with a new kind of “animal”. This is demonstrated in part by the breadth of examples of the application of AI in this space, where their applications range from recommendation engines to supply chain management and fraud detection models. Furthermore, the technology and its possibilities are evolving quickly – a great example is the abilities of Siri and how it has evolved. For these reasons, thinking creatively to come up with possibilities can be a challenge in itself. Even when considering an established product, the uncertainty arising from the idiosyncrasy of the problem and data adequacy means there is an element of uncertainty about what can be done.
Design thinking and other innovative techniques are the best tools to deal with these challenges in my experience, however, they need to be modified in order to be applied successfully, as AI has unique challenges as a technology. For example, even with perfect data, you may be unsure of the predictive power of the algorithm until you test it out, and small sample user testing can be difficult as the system adapts to each user and context. Getting the right team is also critical.
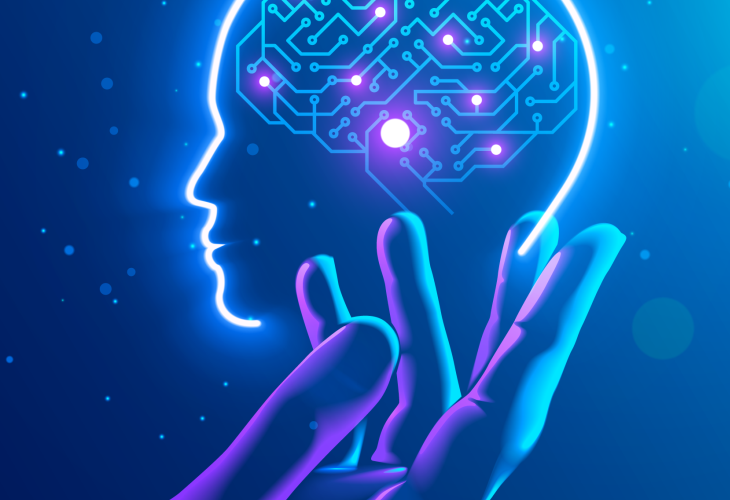
Skills and collaboration
Exploiting AI to its full potential in creating customer experiences that delight customers, create business value, and exploit the full potential of AI, requires multidisciplinary teams to collaborate. The most effective teams, in my experience, are those comprised of: data scientists and engineers – who can harness the power of data and technology reliably and effectively; product designers – who can empathize with customers’ pain points and creatively design features/products and experiences which solve these; and business people – who can evaluate the financial viability of the project and how the proposed product or service will be operationalized by the business. Of course, many other skill sets are required as well.
Projects tend to have a higher failure rate, in my experience, when teams have solely one skillset, for example, a data science team given a mandate to “do something innovative”. Many companies do not use such multidisciplinary innovation teams, and even when they have, these teams find it challenging to align their purpose and communicate effectively.
Privacy and other ethical concerns
Using intelligent systems for any application that affects people – like customer experience – raises important ethical questions that need careful consideration. Two of the biggest are dealing with data privacy and algorithmic bias (The Montreal Declaration provides a broad framework that goes beyond these for those interested).
Recent regulation has gone some way to address the privacy concern in some jurisdictions (e.g., the introduction of GDPR in Europe), forcing companies to compromise on the AI model and data being used. In the future federated learning (where algorithms may be trained in a distributed way, on-device) and homomorphic encryption (being able to use machine learning on encrypted data preserving privacy) will play a key role in providing privacy while still enabling AI.
Bias is much more difficult and tricky to deal with because unbiased data is nearly impossible to find, and even if it were possible, there is no unique technical definition for being unbiased/fair, meaning practitioners have to make trade-offs as they will not be able to get all the forms of fairness that they want. Companies, therefore, require an ethical framework to work within and ensure their algorithms live up to their values.
Governing and validating the use of artificial intelligence on an enterprise scale is challenging and not something that many organizations that have recently implemented AI have grappled with to date.
There are several challenges, the main ones being: identifying all uses of AI across the enterprise, dealing with volumes of data in validation teams, explaining models and results to stakeholders and customers, tracing data back to the source (especially to look through feature engineering), and validating hyper-parameters (which are often educated guesses).
Data challenges
Data is possibly the biggest challenge that most organizations face when trying to implement artificial intelligence. The quality and quantity of data can often prohibit applications of AI that would otherwise be possible. Even where data has been collected perfectly, it can be challenging when there is significant volume or where the data needs to be aggregated and reconciled across several systems.
A large volume of data in certain circumstances (e.g. sensor data perhaps from telematics devices installed in cars to monitor drivers of fleets or to price insurance can be a significant challenge to transmit, warehouse, and manipulate). Being good at sourcing data (both internally and externally), merging it usefully, and dealing with data that is less than ideal is table-stakes to work in innovative AI spaces. Newer technologies such as ‘data fabrics’ will play a bigger role in enabling this in the future.
Conclusion
In summary, firms should adopt a broad view recognizing that there are many facets to customer experience and many functions within a firm that affect it. In this context, AI has four major roles to play in improving how customers experience the products and services they use: measuring customer experience in real-time and providing deep comprehensive insights into customer experience, building smart, personalized, and contextually aware products and services, improving back-end operations which then impact customer experience, and providing designers and innovators with new tools for customer experience design.
The challenges here include innovating with AI as thinking of use cases can be unintuitive and challenging, getting multidisciplinary teams of data scientists, designers, and business managers to collaborate well, dealing with ethical issues such as bias and privacy, and, of course, dealing with poor and inadequate data. However, for those willing to take on these challenges, AI opens up new yet undiscovered possibilities for winning the hearts and minds of customers!
Disclaimer: All views and opinions in this article are the author’s and do not relate to those of their employer.